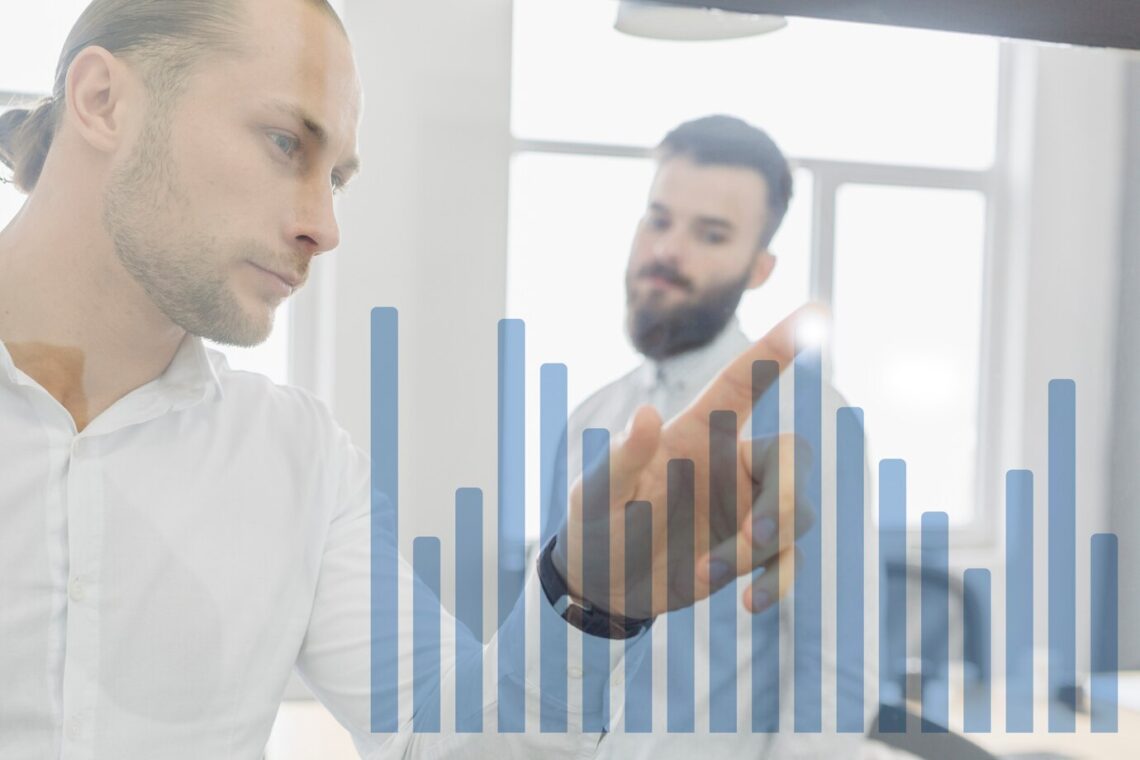
Advanced Technical Indicators: Beyond the Basics
Moving averages and MACD oscillators are like training wheels for forex traders. But once you’ve mastered the basics, it’s time to shed the stabilisers and explore the vast terrain of advanced technical indicators. This article unveils sophisticated tools like Elliott Wave Theory and Fibonnaci Retracements, empowering you to decipher complex market patterns and predict price movements with laser-like precision.
Background Information
The roots of technical analysis can be traced back to the Dow Theory in the late 19th century, where Charles Dow laid the foundation for analyzing price movements to predict future market trends. Over time, basic technical indicators like moving averages, MACD (Moving Average Convergence Divergence), and RSI gained popularity for their simplicity and effectiveness in identifying trend reversals and potential entry/exit points. These indicators became staples for traders and investors seeking to gain insights from historical price data.
As financial markets evolved and technology advanced, the need for more sophisticated analysis tools became apparent. The increasing complexity of global markets, the rise of algorithmic trading, and the influx of big data necessitated a shift towards advanced technical indicators capable of capturing subtleties in market behavior. Traders and analysts sought tools that could offer a deeper understanding of price movements and better guide decision-making in an increasingly dynamic and interconnected financial landscape. The demand for these advanced tools prompted the development and integration of more complex indicators into technical analysis methodologies.
Advanced Technical Indicators
- Ichimoku Cloud: One of the advanced technical indicators making waves in modern trading is the Ichimoku Cloud. It comprises multiple components, including the conversion line, base line, and cloud, providing a comprehensive view of potential support, resistance, and trend strength. Traders can assess not only the direction of the trend but also its momentum and key levels, offering a more nuanced perspective than traditional indicators.
- Bollinger Bands %B: Building upon the classic Bollinger Bands, the Bollinger Bands %B indicator adds a layer of sophistication by indicating where the current close lies within the bands. This allows traders to identify overbought or oversold conditions more precisely, enhancing the ability to anticipate potential reversals or trend continuations.
- Fibonacci Extensions: While the Fibonacci retracement tool is a staple in technical analysis, advanced traders often incorporate Fibonacci extensions for a more comprehensive analysis. These extensions provide additional price levels beyond the standard retracement levels, aiding traders in identifying potential price targets and gauging the strength of a trend.
These advanced indicators represent a departure from the simplicity of basic tools, offering traders a more intricate understanding of market dynamics. As financial markets continue to evolve, the adoption of these advanced indicators becomes crucial for those aiming to stay ahead of the curve and make more informed trading decisions.
Sentiment Analysis
Understanding market sentiment is paramount in today’s fast-paced trading environment. As markets are not solely driven by numerical data but also by the collective psychology of participants, advanced technical analysis has incorporated sentiment analysis. This involves assessing the prevailing mood among traders and investors, often derived from sources such as social media, news articles, and financial forums.
Fear and Greed Index
One of the tools employed in sentiment analysis is the Fear and Greed Index, which quantifies the overall sentiment in the market. By evaluating factors like volatility, market momentum, and put/call ratios, this index provides a snapshot of whether the market is driven by fear or greed. Incorporating sentiment analysis into technical indicators offers traders an additional layer of information to complement traditional metrics, helping them gauge the emotional aspect of market participants and make more informed decisions.
Machine Learning in Sentiment Analysis
The integration of machine learning techniques further refines sentiment analysis. Algorithms can sift through vast amounts of textual data, extracting patterns and discerning shifts in sentiment over time. Machine learning models can adapt to changing market conditions, providing a more dynamic understanding of how sentiment influences price movements. The combination of sentiment analysis and machine learning adds a sophisticated dimension to technical analysis, allowing traders to factor in the human element of trading.
Machine Learning in Technical Analysis
As financial markets become increasingly data-driven, the integration of machine learning (ML) in technical analysis has emerged as a powerful tool for traders and investors. ML algorithms have the ability to process vast datasets, identify intricate patterns, and adapt to changing market conditions, providing a level of analysis beyond the capabilities of traditional indicators.
Incorporating ML into technical analysis often involves the use of algorithms like neural networks, decision trees, and support vector machines. These algorithms are trained on historical market data, learning from patterns and relationships to make predictions about future price movements. The adaptability of ML models allows them to continuously evolve as market dynamics change, providing traders with a more dynamic and responsive approach to technical analysis.
Example of Machine Learning Models in Technical Analysis
Model | Description | Applications |
Neural Networks | Mimic the human brain, learning complex patterns. | Pattern recognition, trend analysis. |
Decision Trees | Tree-like structures for decision-making based on input features. | Decision points, scenario mapping. |
Support Vector Machines | Classify data points into different categories, useful for trend identification. | Trend classification, market condition analysis. |
Explanation of the Table:
- Neural Networks: These models, inspired by the human brain, excel at recognizing complex patterns in data. In technical analysis, neural networks are used to identify intricate relationships between various market factors, enabling more accurate predictions.
- Decision Trees: Decision trees are tree-like structures that make decisions based on input features. In technical analysis, these models are employed to map out potential decision points in the market, aiding traders in navigating complex scenarios.
- Support Vector Machines (SVM): SVM is a classification algorithm that categorizes data points into different groups. In technical analysis, SVM can be used to identify trends and classify market conditions, assisting traders in making more informed decisions.
While machine learning in technical analysis offers promising benefits, it is not without challenges. Overfitting, the risk of tailoring a model too closely to historical data, remains a concern. Additionally, the interpretability of ML models can be limited, making it crucial for traders to combine these advanced techniques with a solid understanding of fundamental analysis for more robust decision-making.
Limitations and Criticisms
Despite the advancements brought about by advanced technical indicators and the integration of machine learning in technical analysis, there exist notable limitations and criticisms that traders and analysts should consider.
One significant concern is the risk of overfitting. Machine learning models may perform exceptionally well on historical data used for training but may struggle to generalize to new market conditions. Overfitting occurs when a model becomes too tailored to historical data, capturing noise rather than genuine patterns. Traders employing machine learning techniques must be vigilant in ensuring that their models are robust and capable of adapting to evolving market dynamics.
Another criticism revolves around the inherent assumption of repeating historical patterns. While technical analysis relies on the idea that historical price movements can predict future trends, financial markets are dynamic, and past performance does not guarantee future results. This critique emphasizes the importance of incorporating a balance of technical and fundamental analysis to obtain a more comprehensive understanding of an asset’s potential.
Moreover, the inclusion of subjectivity in sentiment analysis introduces an additional layer of complexity. Interpreting market sentiment from sources like social media or news articles can be challenging, as it often involves qualitative assessments. Traders must recognize the potential for misinterpretation and carefully consider the reliability of sentiment indicators in their decision-making process.
A broader criticism concerns the potential detachment from fundamental analysis. While advanced technical indicators and machine learning models provide sophisticated tools for predicting price movements, they may not always account for the underlying health and value of an asset. Relying solely on technical analysis may lead to a lack of awareness regarding broader economic factors, potentially exposing traders to unforeseen risks.

